Unleashing the Power of Data Annotation: How to Annotate Image Online Efficiently
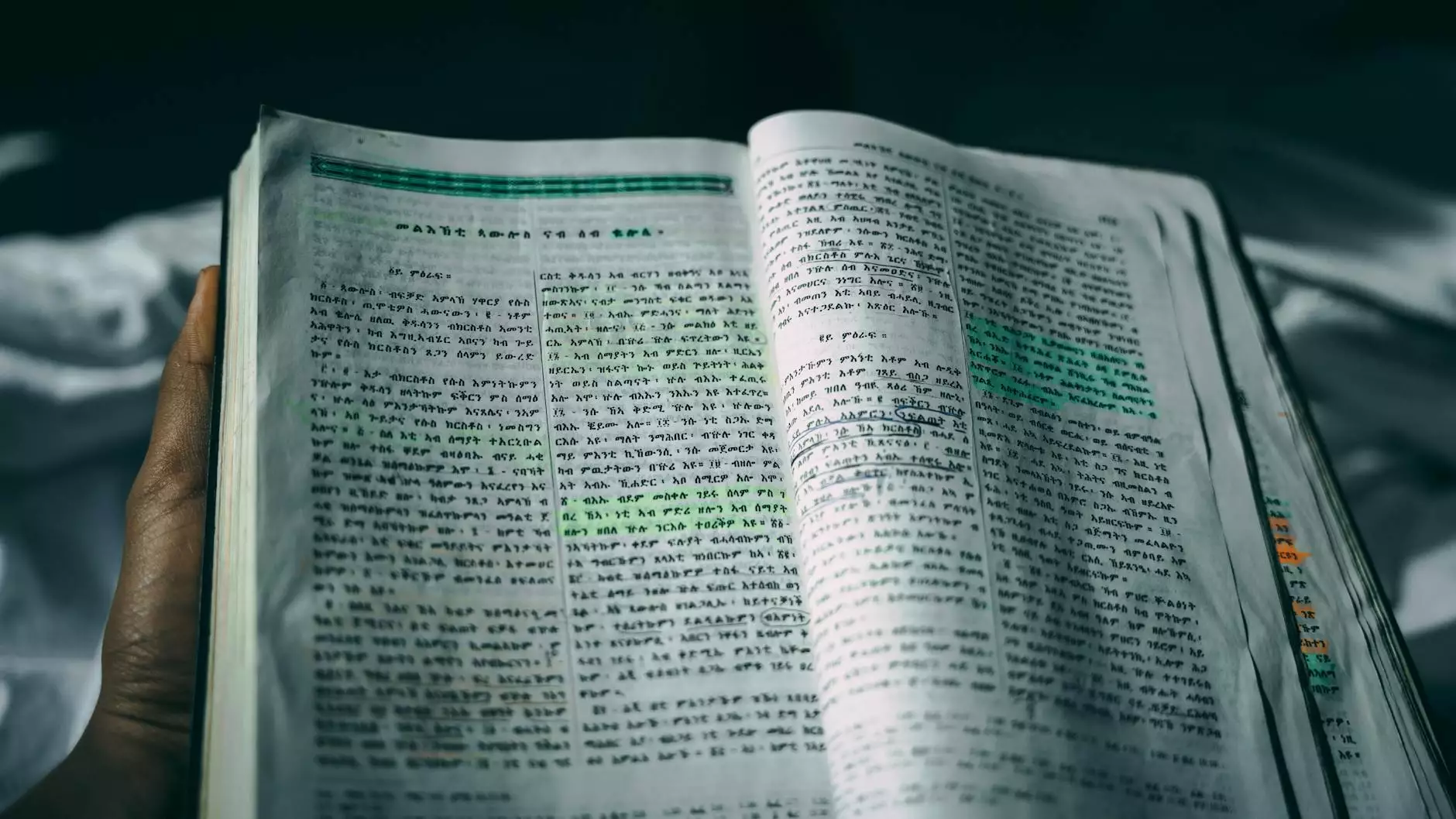
In the rapidly advancing world of technology, the ability to annotate images online has become an indispensable skill for businesses looking to leverage data for machine learning and artificial intelligence (AI) projects. Whether you're building a complex model for automated perception systems or enhancing user experiences through data-driven insights, understanding how to manage and utilize data annotation tools effectively is paramount.
The Importance of Data Annotation
Data annotation serves as the foundation for supervised learning in machine learning algorithms. By providing structured data through annotation, machines can learn from patterns and make predictions or decisions based on that data. Here are some reasons why data annotation is crucial:
- Enhances Model Performance: An algorithm trained on well-annotated data performs substantially better than one trained on poorly labeled data.
- Facilitates Accurate Predictions: Annotated data allows AI models to learn from context, improving their ability to make accurate decisions and predictions.
- Supports Diverse Applications: From self-driving cars to healthcare diagnostics, data annotation is critical across various sectors.
- Improved Data Understanding: Annotating data not only helps models learn but also helps teams understand the datasets better.
What is Image Annotation?
Image annotation involves labeling or tagging images with informative context that enables machine learning models to understand and recognize the content of the images. This process is crucial for training various computer vision applications such as object detection, facial recognition, and image segmentation.
There are several types of image annotation, including:
- Bounding Box Annotation: This method involves drawing rectangular boxes around objects of interest in an image to identify their location.
- Polygon Annotation: More intricate than bounding boxes, polygon annotation involves outlining the shape of an object with multiple vertices for precise identification.
- Semantic Segmentation: This technique classifies each pixel in an image, providing a comprehensive understanding of the image's content.
- Keypoint Annotation: Used mainly in facial recognition, this involves marking specific features of an object, like eyes, nose, and mouth.
How to Annotate Image Online Efficiently
With the rise of various data annotation platforms, learning how to annotate images online has never been easier or more efficient. Here’s a guide on how to get started:
1. Choose the Right Tool
The first step in your online image annotation journey is selecting a robust tool. Look for features such as:
- User-Friendly Interface: The tool should be easy to navigate.
- Collaboration Features: If you're working in teams, look for tools that enable multiple users to annotate images collaboratively.
- Integration Capabilities: Ensure the tool can integrate with your existing workflows or machine learning frameworks.
- Support for Various Annotation Types: Choose a tool that supports different annotation styles as needed by your project.
2. Set Up Your Project
After choosing your tool, the next step is to set up your project. This involves:
- Uploading Images: Import the images you wish to annotate into the platform.
- Defining Annotation Guidelines: Provide clear instructions for annotators to ensure consistency.
- Assigning Roles: If teams are involved, assign roles and responsibilities for annotation tasks.
3. Annotate Images
Begin the annotation process by following these actions:
- Select Annotation Type: Decide on the type of annotation required (bounding box, polygon, etc.).
- Tag Relevant Objects: Carefully annotate the objects in the images as per your guidelines.
- Review and Edit: After initial annotations, review and make necessary edits to enhance accuracy.
4. Quality Assurance
Quality assurance is a critical step in the data annotation process. Implement mechanisms for:
- Regular Review: Have seasoned annotators review the work of novices.
- Feedback Loops: Create a feedback system for continuous improvement.
- Statistics Tracking: Monitor annotation progress and quality through key performance metrics.
5. Export the Annotations
Once the images are annotated and quality checks are complete, it’s time to export the annotated dataset. Pay attention to:
- Export Formats: Ensure the exported dataset meets the format requirements of your AI model (e.g., JSON, CSV).
- Data Privacy: Adhere to relevant data privacy regulations when handling datasets.
Why Choose Keylabs.ai for Data Annotation?
At Keylabs.ai, we understand the complexities involved in image annotation. Our data annotation platform provides cutting-edge tools and features that optimize the annotation process, ensuring accuracy and efficiency at every stage. Here’s why we stand out:
- Robust Tools: Our platform boasts a suite of powerful tools designed for diverse annotation needs.
- Expert Support: Our team of experts is dedicated to providing support throughout your annotation journey.
- Scalability: From small projects to large-scale datasets, our platform can scale according to your needs.
- Security: We prioritize data security and privacy, ensuring your data is handled with utmost care.
Common Challenges in Image Annotation
Despite the advantages, businesses often face challenges in image annotation, such as:
- Time Consumption: Annotating large datasets can be time-intensive and laborious.
- Consistency Issues: Maintaining consistent annotation across different teams can be challenging.
- Quality Control: Ensuring that all annotations meet the expected accuracy requires diligence and effective quality control processes.
Future of Data Annotation in Business
The future of data annotation is bright and evolving rapidly. As machine learning and AI continue to advance, the demand for high-quality annotations will only increase. Businesses that prioritize effective annotation strategies will stay ahead of the competition. Here are some trends to watch out for:
- Automation: The automation of the data annotation process through AI-assisted tools can drastically reduce the time and effort needed for annotation.
- Real-time Collaboration: Enhanced collaboration features using cloud-based platforms will facilitate real-time teamwork among annotators globally.
- Open Source Tools: The rise of open-source tools will democratize access to high-quality annotation platforms, fostering innovation.
Conclusion
Annotating images online is a critical component in the data preparation process for AI and machine learning projects. By utilizing effective data annotation tools and strategies, businesses can enhance the performance of their AI models, streamline operations, and improve decision-making processes. At Keylabs.ai, we provide comprehensive solutions tailored to meet your unique annotation needs, ensuring that your data is not just annotated but accurately and efficiently labeled.
In conclusion, as businesses increasingly rely on data to drive their operations, mastering the art of data annotation will empower organizations to unlock new opportunities, innovate processes, and deliver exceptional results in a competitive landscape.